Revolutionizing Music Genre Classification with Advanced Audio Representations
In the dynamic field of Music Information Retrieval (MIR), traditional approaches have largely depended on Mel spectrograms, a time-frequency-based audio representation that effectively captures essential audio features. However, with the introduction of new AI models like Jukebox, there’s potential to revolutionize how we perceive and categorize music. A study by Navin Kamuni and colleagues explores a novel audio representation method using deep vector quantization, proposed by the Jukebox model, for the identification of music genres. This representation was pitted against traditional Mel spectrograms, using a comparably sophisticated transformer design but trained on a modest dataset of 20,000 tracks.
The results were intriguing; the Jukebox model did not surpass the performance of Mel spectrograms. This could indicate that Jukebox’s representation, while innovative, may not capture the nuances of human auditory perception as effectively as Mel spectrograms, which are specifically designed with this in mind. This study underscores the importance of tailored audio representations that align with the complexities of human hearing, suggesting a potential for further refinement and exploration in future MIR applications.
Reference: Kamuni, Navin, et al. “A Novel Audio Representation for Music Genre Identification in MIR.” arXiv preprint arXiv:2404.01058 (2024).
AI and ML in Audio Fingerprinting: Addressing Real-World Challenges:
Audio fingerprinting technology, exemplified by pioneers like Shazam, has transformed how we identify and interact with music. Despite its successes, current audio fingerprinting systems struggle with accuracy in noisy or distorted environments. A recent study spearheaded by N. Kamuni proposes an integrated AI and ML approach to enhance the robustness of audio fingerprinting algorithms. Based on the Dejavu Project’s framework, this research focuses on improving system accuracy in real-world conditions by simulating various background noises and distortions.
The study’s methodology incorporates advanced signal processing techniques, including the Fast Fourier Transform, spectrograms, and an innovative use of the “constellation” concept for peak extraction and fingerprint hashing. This approach led to a notable performance leap, achieving 100% identification accuracy within five seconds of audio input, which represents a significant advancement in the field. This research not only demonstrates the potential of AI and ML to enhance audio recognition technologies but also highlights the practical implications of these technologies in diverse and challenging auditory environments.
Reference: N. Kamuni, S. Chintala, N. Kunchakuri, J. S. A. Narasimharaju and V. Kumar, “Advancing Audio Fingerprinting Accuracy with AI and ML: Addressing Background Noise and Distortion Challenges,” 2024 IEEE 18th International Conference on Semantic Computing (ICSC), Laguna Hills, CA, USA, 2024, pp. 341-345, doi: 10.1109/ICSC59802.2024.00064.
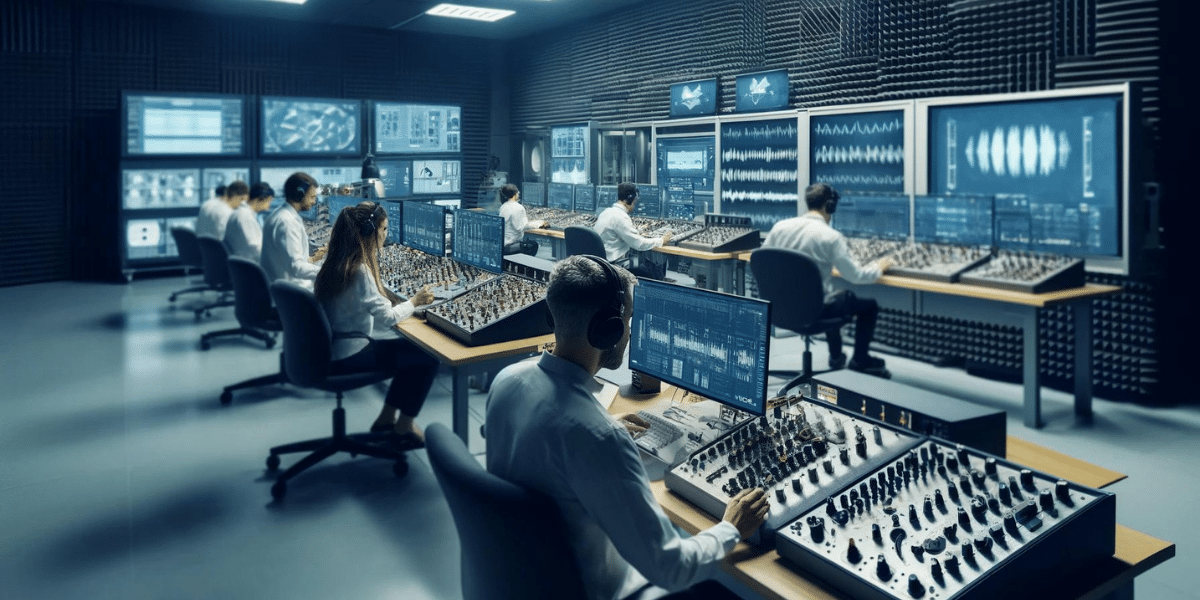
Photo Courtesy: Jukebox
Emerging Use Cases and Future Applications
The implications of these advancements are vast and varied. In the world of smart home technology, more robust audio fingerprinting can enhance voice assistant responsiveness and accuracy in noisy environments, leading to more intuitive user interactions. In the security sector, improved audio recognition can facilitate more reliable surveillance systems capable of detecting distinct sounds or voices in high-noise backgrounds.
Another promising application is in healthcare, where advanced audio representations could be used for monitoring and diagnosing conditions through the analysis of voice and breath sounds, potentially identifying patterns that precede medical events such as asthma attacks or episodes of sleep apnea.
Additionally, the entertainment industry could leverage these technologies to offer more immersive and personalized experiences. For example, by identifying the genre of music or specific songs playing in a user’s environment, services could recommend content that aligns with the user’s current activity or mood, enhancing engagement and satisfaction.
These studies and their potential applications not only highlight the importance of continuous innovation in audio processing but also illustrate the transformative impact of AI and ML on our interaction with technology in everyday life. As these technologies evolve, they promise to refine our digital experiences, making them more seamless and responsive to our human needs.
Published By: Aize Perez